-
π Hi, Iβm Teo Wu (officially Haoning Wu), working on LMMs in Rhymes AI, closely advised by Dongxu Li and Junnan Li. Prior to this, I have been a PhD candidate (preparing thesis defense) in Nanyang Technological University πΈπ¬, supervised by Prof. Weisi Lin. I obtained by B.S. degree of computer science in Peking University (εδΊ¬ε€§ε¦).
-
I am currently focusing on LMM pre-training and evaluation (video & longer context & better instruction-following). See our LongVideoBench, the first video benchmark for LMMs proven improvable given more input frames (>=256). I have also contributed to video and long-context training of Aria (Model, Paper, GitHub), an excellent open-source native MoE LMM with abilities matching GPT-4o-mini/Gemini-1.5-Flash in only 3.9B activated parameters.
-
π± I have also been the lead of project Q-Future: Visual Evaluation with LMMsπΉ, on which 7 first-authored papers accepted in top conferences and journels including ICML, ICLR, NeurIPS, TPAMI, CVPR, ECCV and ACMMM. The flagship scorer, OneAlign has been downloaded more than 238K times (until Jul 25, 2024) on HuggingFace.
-
Prior to LMMs, my PhD topic was on video quality assessment, a traditional area trying to gauge the quality scores (and more) on videos. Among 6 papers published in that area (in ECCV, ICCV, TPAMI, etc), the two representative works are FAST-VQA and DOVER, which have been most-used baselines in that field.
- π« Reach me by e-mail: realtimothyhwu@gmail.com/haoning001@e.ntu.edu.sg, Twitter: Twitter
- Google Scholar
π₯ News
- 2024.10.11: Β ππ We release Aria, a native LMM that excels on text, code, image, video, PDF and more!
- 2024.09.26: Β ππ LongVideoBench get accepted by Neurips2024 D&B track!
- 2024.09.26: Β ππ Compare2Score get accepted by Neurips2024 as Spotlight!
- 2024.08.08: Β ππ Extension of Q-Bench (Q-Bench+, on image pairs) get accepted by TPAMI!
- 2024.07.16: Β ππ 4 papers in Q-Future get accepted by ACMMM (1 first-authored, 3 Oral)!
- 2024.07.02: Β ππ Co-Instruct get accepted by ECCV2024 as an Oral paper!
- 2024.05.02: Β ππ Q-Align get accepted by ICML2024 (score: 7765)!
- 2024.02.27: Β ππ Q-Instruct get accepted by CVPR2024!
- 2024.01.16: Β ππ Q-Bench get accepted by ICLR2024 as a Spotlight paper!
- 2023.09.10: Β ππ Extension of FAST-VQA (FasterVQA) get accepted by TPAMI!
- 2023.07.26: Β ππ MaxVQA get accepted by ACMMM2023 as an Oral paper!
- 2023.07.14: Β ππ DOVER get accepted by ICCV2023!
- 2023.02.28: Β ππ DisCoVQA get accepted by TCSVT. First paper written in my PhD career.
- 2022.07.07: Β ππ FAST-VQA get accepted by ECCV2022!
Projects
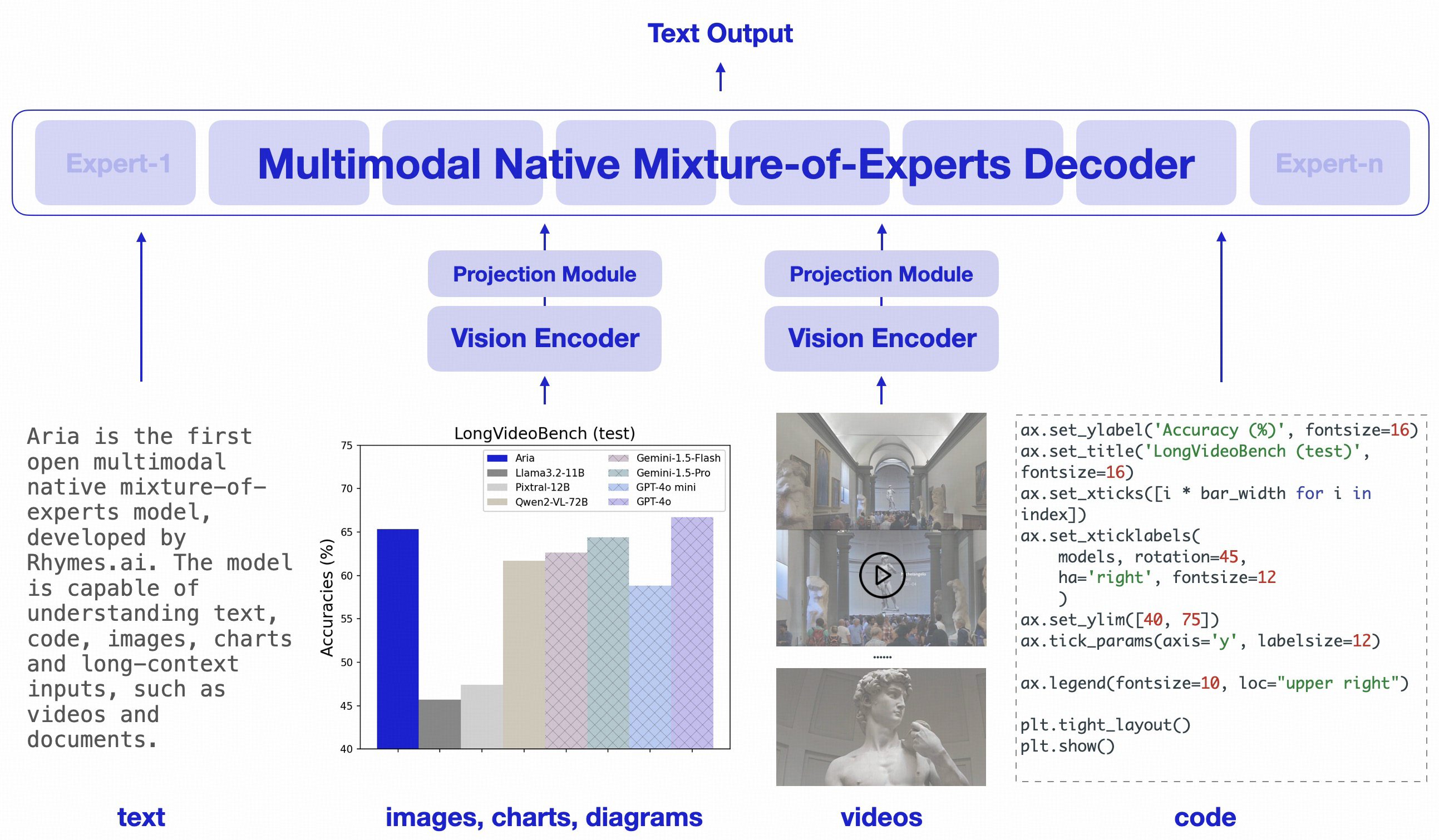
Aria: First Open Multimodal Native MoE Model
- The first native multi-modal MOE model, excels on text, code, image, video, PDF, etc.
- Extraordinary 64K context length, can steadliy improves on long video benchmarks until 256 input frames.
- #2 on LongVideoBench (#1 open-source), #6 on VideoMME (#1 open-source <70B parameters), #3 on MMBench-Video (#1 open-source), #1 on MLVU-Test, all evaluated under 256 uniform frames.
π Selected Publications
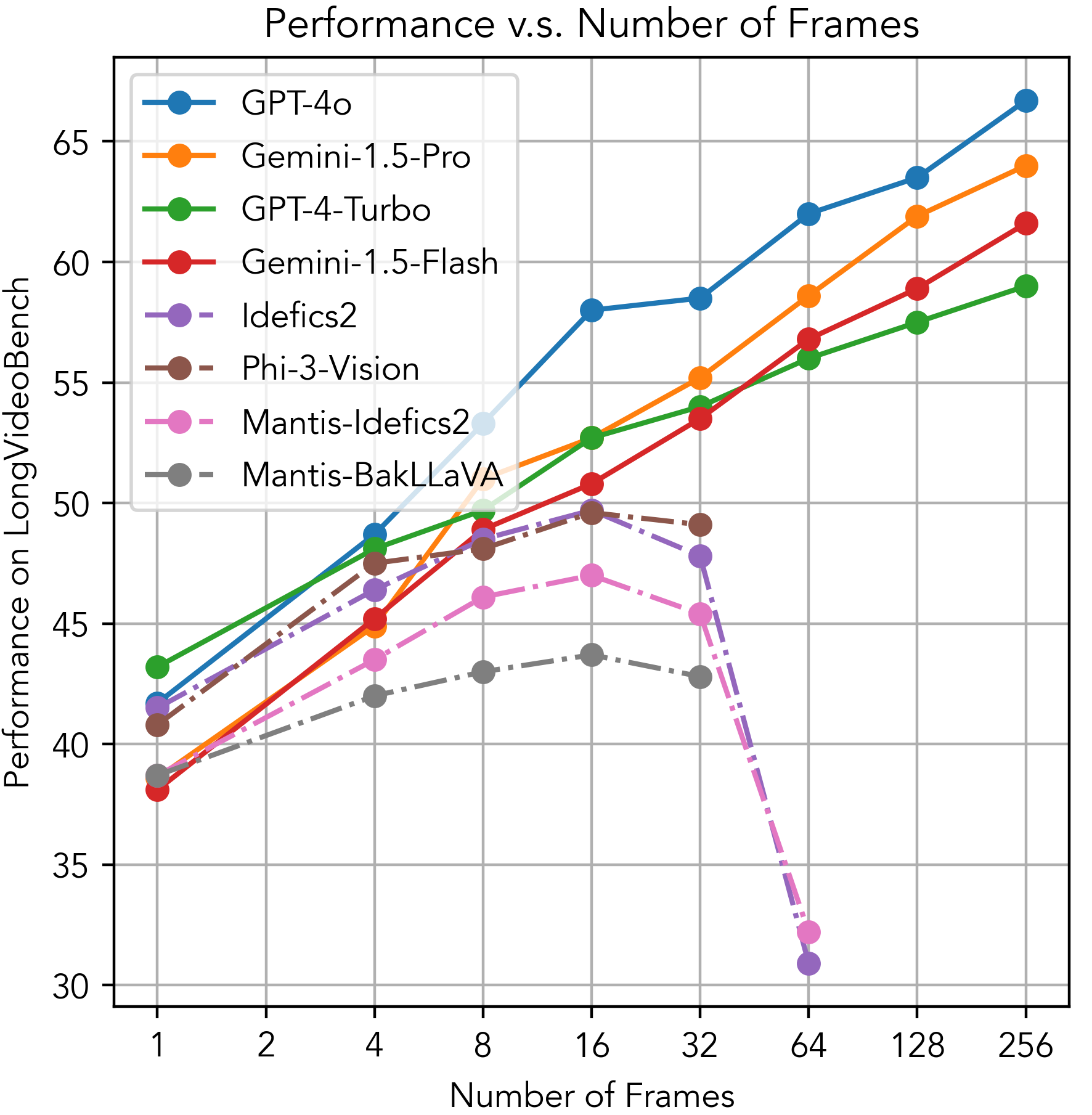
LongVideoBench: A Benchmark for Long-context Interleaved Video-Language Understanding
- Authors: me, Dongxu, Bei Chen, Junnan
- TL, DR: A benchmark for long videos that truly requires a long input (i.e. hundreds of frames). First-ever non-synthetic benchmark (6.7K human-crafted MCQs) on long-context multi-modal understanding.
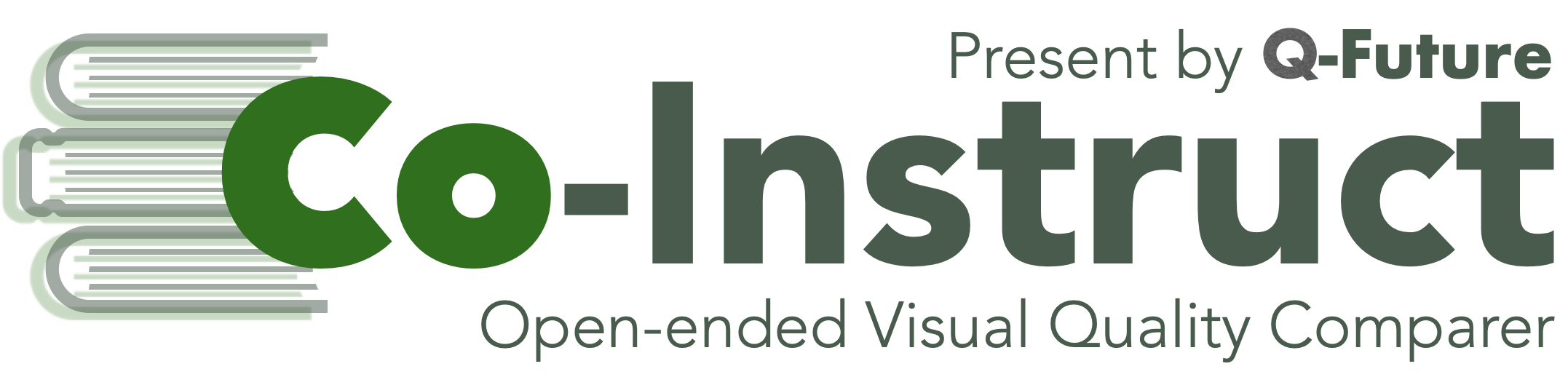
Towards Open-ended Visual Quality Comparison
- Authors: me, Hanwei, Zicheng, et al.
- TL, DR: The first work for MLLMs on visual quality comparison, via bootstrapping human and LLM, plus distilling GPT-4v. It is a very early attempt on multi-image MLLM. Proposed dataset has been integrated by LLaVA-Interleave and Mantis.
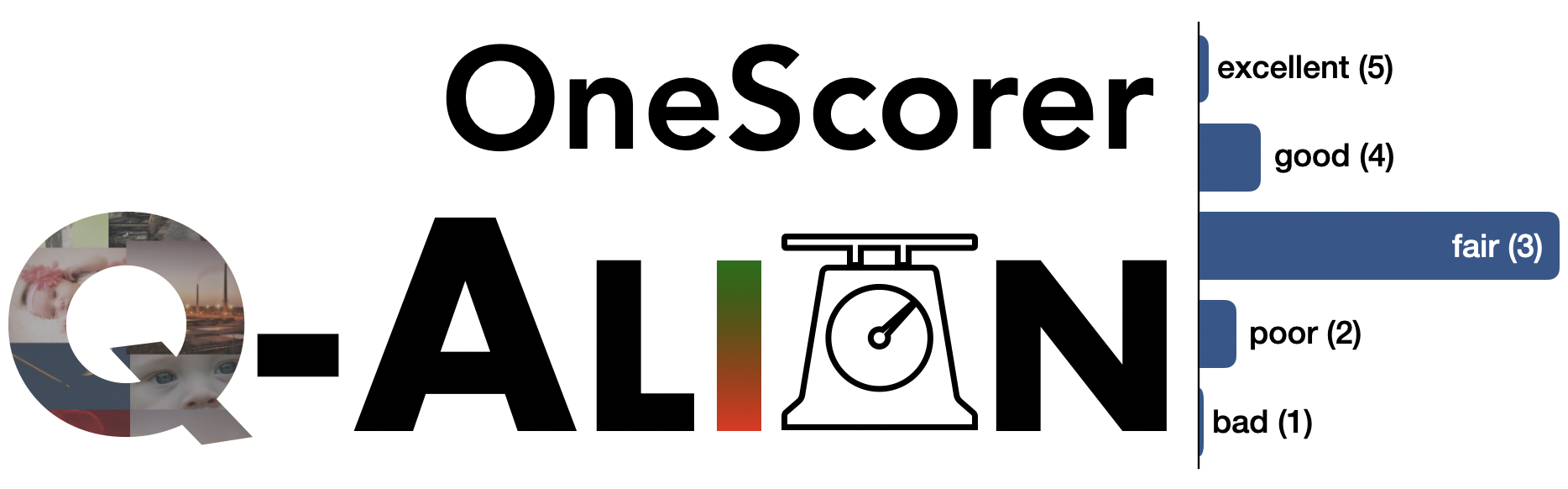
Q-Align: Teaching LMMs for Visual Scoring via Discrete Text-Defined Levels
- Authors: me, Zicheng, et al.
- TL, DR: The best visual quality and aesthetic scorer so far (disclaimer: until Jul 25, 2024); Yet a better way to train LMMs on scoring. It fine-tunes an LMM with discrete text levels (good, poor, fair) and extract the weighted average of token probabilities for continuous scores on inference. SOTA on 12 datasets.
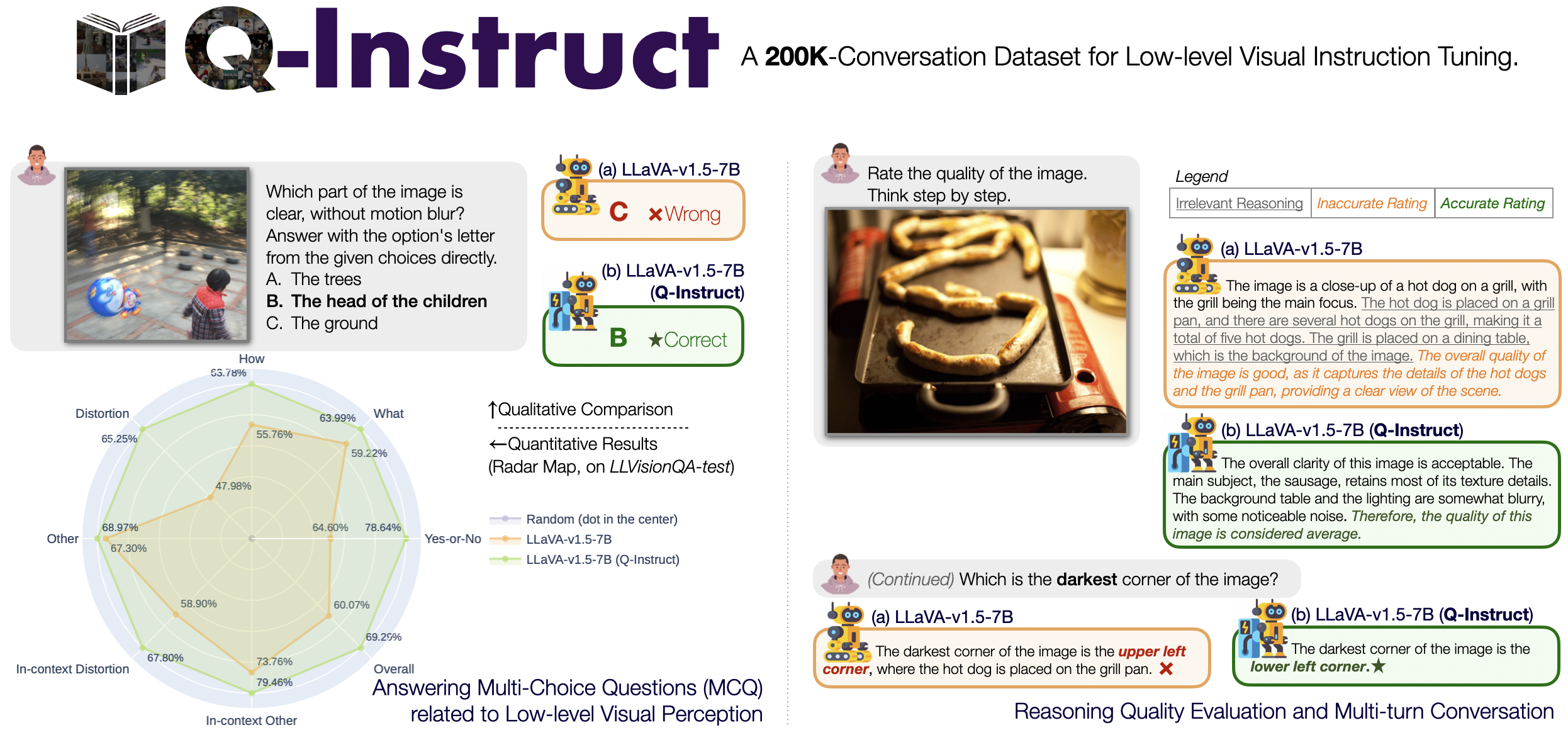
Q-Instruct: Improving Low-level Visual Abilities for Multi-modality Foundation Models
- Authors: me, Zicheng, Erli, et al.
- TL, DR: The first low-level visual instruction tuning dataset. Includes GPT to generate QA pairs based on human-annotated quality descriptions.

Q-Bench: A Benchmark for General-Purpose Foundation Models on Low-Level Vision
- Authors: me, Zicheng, Erli, et al.
- TL, DR: The first-things-first of Q-Future, defining three important tasks: low-level description (similar to captioning), low-level question-answering, and scoring. It has been extended to multi-image versions (Q-Bench+) as well as on AI-generated artifacts (A-Bench).